Managing AI Projects: Key Challenges and Solutions for Product Managers
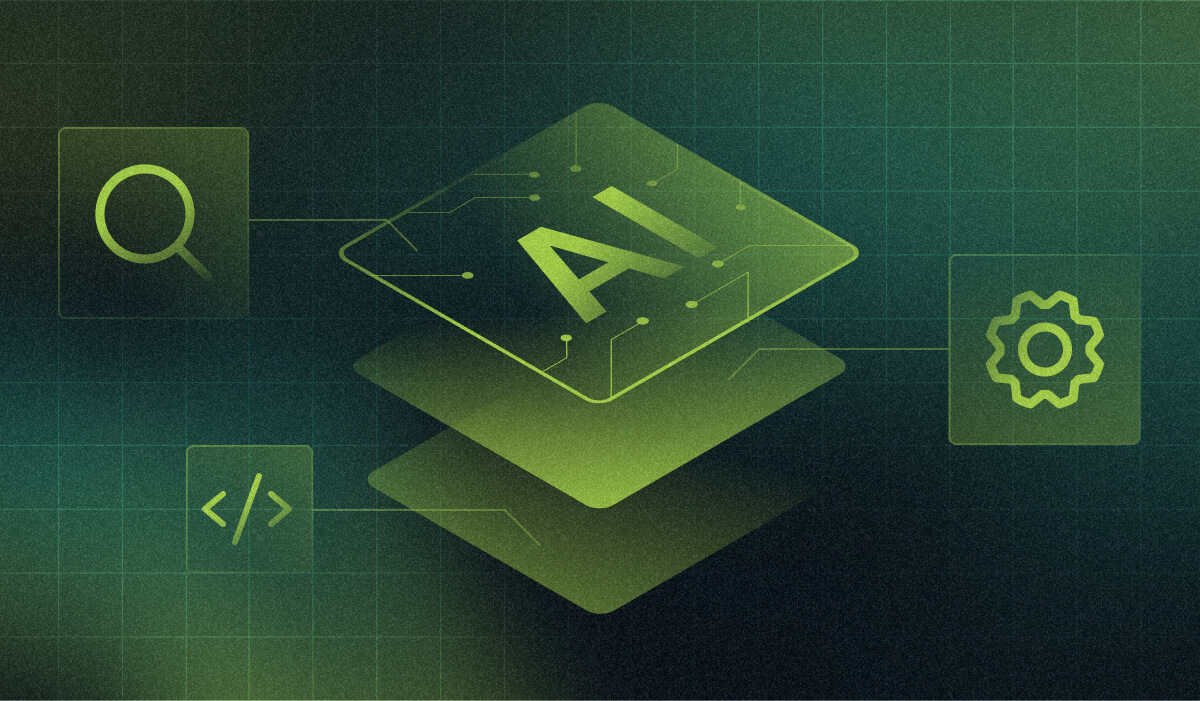
As product managers, we are constantly faced with new challenges and emerging technologies. The role of product managers is largely fluid as it is. And as expectations are always increasing, it’s essential to continue to develop a fundamental understanding of how to correctly leverage AI and ML.True - as both expectations and regulations change over the coming years, uncertainty will undoubtedly still surround many AI initiatives. Which is why having the ability to keep learning and making incremental improvements is critical going forward.
But hey, challenges are meant to be conquered.
In this article, we will explore the key challenges faced by product managers in AI projects and provide solutions to navigate these complexities successfully.
Understanding the Scope and Potential of AI
Challenge: The vast scope of AI can be overwhelming for product managers. It is essential to grasp the potential and limitations of AI technologies to effectively leverage them in projects.
Solution: Invest time in understanding the fundamentals of AI and its various subfields, such as machine learning and natural language processing. Collaborate with AI experts within your organization or seek external guidance to gain insights into the capabilities and applications of AI. This knowledge will enable you to make informed decisions and set realistic expectations for AI projects.
Data Availability and Quality
Challenge: AI heavily relies on large quantities of high-quality data for training models. Acquiring, organizing, and maintaining relevant data can pose significant challenges for product managers.
Solution: Work closely with data engineers and data scientists to identify the required data sources and ensure data availability. Define data quality standards and establish data governance practices to ensure accuracy, completeness, and privacy compliance. Collaborate with cross-functional teams to build robust data pipelines and leverage data augmentation techniques to address data scarcity issues.
Ethical Considerations
Challenge: AI projects raise important ethical considerations, including biases in data, privacy concerns, and potential societal impact. Product managers must navigate these ethical challenges while ensuring responsible and ethical use of AI technologies.
Solution: Foster a culture of ethical AI within your organization. Implement ethical guidelines and review processes to address potential biases in data and algorithms. Engage in ongoing dialogue with stakeholders, data privacy experts, and legal teams to ensure compliance with regulations and protect user privacy. Regularly assess the societal impact of AI applications and take proactive steps to mitigate potential risks.
Interpreting and Explaining AI Results
Challenge: AI models often produce complex and opaque outputs, making it difficult for product managers to interpret and explain the results to stakeholders and end-users.
Solution: Collaborate closely with data scientists and AI experts to develop explainable AI models and interpretability techniques. Leverage visualization tools and techniques to present AI results in a clear and understandable manner. Engage in effective communication with stakeholders, providing context, insights, and limitations of AI-generated outputs to build trust and facilitate decision-making.
Agile Development and Iterative Learning
Challenge: AI projects often require an iterative approach, where learning and refinement occur throughout the development process. Balancing agile development methodologies with long training cycles and evolving requirements can be a challenge for product managers.
Solution: Embrace agile project management methodologies while adapting them to the unique characteristics of AI projects. Break down AI initiatives into smaller manageable components or use cases, enabling rapid feedback cycles and continuous learning. Implement mechanisms to incorporate user feedback, iterate on models, and adapt to evolving requirements.
Key takeaways
It is unlikely that companies are going to be hiring people for AI product manager roles outright; there simply aren’t enough people in the world with experience managing AI projects in the enterprise.
Instead, many existing product managers at large companies will find themselves in charge of AI products in the new decade.
That’s why it is so important to prepare yourself to navigate a unique set of challenges and embrace new approaches.
By understanding the scope of AI, addressing data-related concerns, upholding ethical considerations, interpreting AI results, and embracing agile methodologies, product managers can effectively lead AI projects to success.