Unleashing the Power of Riva: Revolutionizing Specific Domains with Superior ASR Technology
Did you know that Automatic Speech Recognition (ASR) technology has emerged as a game-changer, revolutionizing the way we interact with machines and boosting productivity?
Today, neural network-based solutions and hidden Markov models dominate the field, showcasing remarkable performance in general domain speech recognition tasks. However, when it comes to real-life conditions with domain-specific vocabulary, the accuracy of ASR systems often takes a nosedive. In this article, we delve into the exciting world of ASR, explore common benchmarks, and reveal how innovative techniques can revolutionize speech recognition in specific domains. Get ready to uncover the exciting secrets behind Riva, a leading ASR solution that sets itself apart with exceptional performance, domain-specific customization, and advanced features.
Benchmarking ASR Systems
When evaluating ASR systems, the word error rate (WER) serves as a typical metric. For general domain data, benchmarks like the TIMIT Acoustic-Phonetic Continuous Speech Corpus and LibriSpeech dataset are commonly used. Facebook's groundbreaking wav2vec model and Google's Conformer network with wav2vec pretraining currently lead the pack in terms of performance.
Wall Street Journal corpus is also used for ASR models evaluation. It is based on the dictations of the WSJ newspaper and contains 80 hours of speech. This benchmark also has 2 versions of test data: eval92 and eval93. For the former, the state-of-the-art is the SpeechStew model, and for the latter it is DeepSpeech 2.
The results for all these datasets are given in “Table 1.”
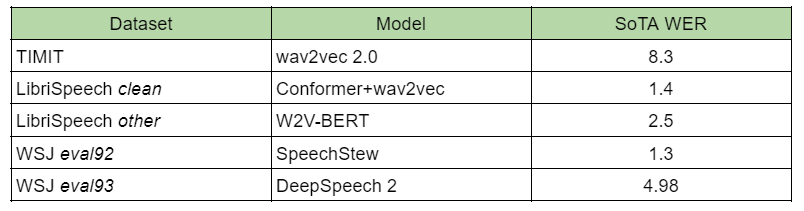
Domain-specific Challenges
While general domain speech recognition achieves over 90% accuracy, the real challenge lies in recognizing domain-specific vocabulary. Applying a general domain ASR system to recordings packed with specialized terminology results in a drastic increase in WER, often exceeding 50%. Out-of-vocabulary (OOV) words are typically replaced with "UNK" tags, while general domain words may replace specific terms, leading to distorted output sequences. This presents a major obstacle for applications requiring recognition of product names or services in call centers or voice chatbots.
Overcoming the Domain-Specific Barrier
Training ASR models on domain-specific data is ideal, but limited data availability or legal constraints often hinder this approach. However, even with scarce data, fine-tuning techniques offer a ray of hope. By leveraging large pre-trained models, which have a strong grasp of general language knowledge, specific words and combinations can be recognized effectively. Fine-tuning mitigates the OOV word issue and significantly reduces the model's WER.
Our Success Story
At our company, we tackled the challenge of enhancing speech recognition for a call center focused on electric equipment. Armed with 11.27 hours of labeled call recordings, we had 7.47 hours for training and 3.8 hours for testing. To evaluate the model's performance accurately, we introduced a new metric: term error rate (TER). We compared NVIDIA Riva's Conformer-CTC model, Facebook's wav2vec, and Google Cloud Speech-to-Text as alternatives.
In “Table 2” and “Table 3,” we present the results of all these models, with both out-of-the-box and fine-tuned versions. “Table 2” stands for the results on the test set provided by the client, and “Table 3” shows the results on the LibriSpeech other test set.


Unleashing the Power of NLP
NVIDIA Riva's Conformer-CTC model proved ideal for our case. Its customizable architecture, GPU optimization, and remarkable out-of-the-box performance made it a standout choice. By fine-tuning the model with our domain-specific corpus and augmenting the vocabulary, we achieved exceptional results. Facebook's wav2vec, another strong contender, demonstrated promising performance after fine-tuning. Meanwhile, Google Cloud Speech-to-Text limited adaptability resulted in a significant WER increase.
The Results
In our tests, the NVIDIA Riva model outperformed both Google and the state-of-the-art solutions for ASR. The fine-tuned Riva model achieved an impressive WER of 10.79%, while wav2vec trailed at 16.23%. On the LibriSpeech other test set, Conformer-CTC and wav2vec2 models showcased their prowess in general speech, while Google Cloud's dramatic WER increase indicated overfitting.
NVIDIA Riva models, backed by fine-tuning and domain-specific training, demonstrate exceptional performance even with limited annotated data. By harnessing the potential of ASR, businesses can unlock a world of possibilities in voice-enabled applications and call center interactions.
Riva's extraordinary impact on specific domains, its ability to enrich ASR with specific domain words, and its unmatched performance make it the undisputed leader in Automatic Speech Recognition.
Embrace Riva today and unlock the true potential of ASR, driving innovation and propelling your business to new heights.